/ News
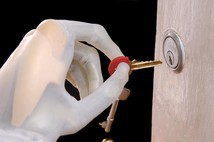
The Problem of Bioprosthesis Control and Possible Solutions
T. Shcukin, "2045" Initiative Coordinator in Russia
Published in collected works of the Nonlinear Dynamics in Cognitive Research 2011 conference, p. 238.
In February 2011, a number of Russian researchers announced plans to create an artificial human body controlled by a neurointerface by the year 2045. Besides the obvious technical difficulties involved in the actual construction of prostheses for limbs and the body, providing an energy source for them, ensuring that their responses to commands are of sufficient duration, and equipping them with sensory elements and feedback capabilities—besides those tasks, the main difficulty is in creating a control system with adequate response capability. Since any control system must be able to receive commands and give feedback, it is logical to state the requirements for the measurement tools within the context of the control system. The term “measurement tools” refers not only to registration of commands from the central nervous system (CNS), but also to the data-processing unit and to a system that searches for patterns among actions taken by the operator of the control system. The term refers, in other words, to the entire range of measurement and processing activities.
Herein we will examine the task that stands before us: An operator must be trained to control a complex system such as a hand prosthesis or even an entire anthropomorphic robot with almost the same number of degrees of freedom as a person. At the same time, it is expected that the control system may differ radically in nature from what we are used to—that is, it may possess a different functional structure, an internal model unfamiliar to the operator. The operator may be asked, for instance, to control a trunk prosthesis or a prosthesis similar to a cephalopod limb.
There are three fundamental approaches to this task (see fig. 1).
Fig. 1. Methods of providing prosthesis control
Commands can be intercepted and re-directed (and, in principle, feedback of various levels from the results of actions can be provided) on different levels of the CNS: both on the level of terminal efferent vessels and on the level of spinal cord nerves, or even on the level of central control. An example of the first approach (block 1.1, fig. 1) is electromyographic control with a whole limb prosthesis (see example [1]) that imitates the movement of a biological hand. An example of the second approach (block 1.3, fig. 1) is experiments in which the control input is activity registered directly from nerve fibers that had formerly innervated a limb (see example [2]). An example of the third approach (block 1.2, fig. 1) is direct electroencephalographic control of a prosthesis (or a computer system) (see example [3]).
In the first two cases, there is practically no training involved, since the systems being used are already functional, having at one time (or even at the same time) exercised control of a limb. The third case is more interesting. In that case there are also two fundamentally different approaches to the task. The first is to use patterns of electroencephalographic (in a broad sense) activity. Initially, those patterns are a representation of activity of functional systems in the brain that send some command to the body. That command is then re-directed to the prosthesis or, more widely, to an artificial body (block 1.2.1, fig. 1). The second is to select arbitrary EEG indices for subsequent use as a state space for the control system (block 1.2.2, fig. 1).
Let’s examine the first option. This kind of approach clearly requires being able to recognize patterns within blocks of maximally sized EEG data and subsequently to map those results onto the entire space of possible states (block 1.2.1.1, fig. 1). The various pattern classes match up with key body commands.
The solution to this problem is complex but well-established. A vocabulary of commands must be built that is equal in number to the quantity of degrees of freedom, or to the quantity of independent variables that form a full map of a system, such as the mechanical system of a wrist prosthesis. After that, we must register the maximum possible number of instances of a command actually being fulfilled in the form of a set of cases. Then, we must conduct pattern recognition, wherein the system of recognizable classes is formed relying on the control system’s space of degrees of freedom, and the set of indicators is formed on the basis of examples of action fulfillment. It is clearly preferable to use programming systems for recognition that are capable of overcoming the problem of combinatorial explosion, working with incomplete input data, and not only learning, as typical artificial neural networks do, but also creating an interpretable set of weighted coefficients, i.e., an “action profile”. It is also desirable to have a built-in statistical-artifact regulation system, as well as a classification system.
In our view, the best approach is software-based, automated systemic cognitive analysis: the “Eidos” system (see example [4]). A set of multidimensional class profiles creates a space that matches the space of degrees of freedom of the system being examined. When the degree of congruity of the real-time data with one or another profile of a previously defined magnitude is increased, a command that matches the profile is delivered to the prosthesis/manipulator.
The set of class profiles aligns with the system coding the movement control system in the registered signals. Since we are working with (for example) EEG, we are referring to the active model of the part of the brain’s functional systems that are reflected in the registered parameters. Knowing these “codes” ahead of time will clearly allow us to significantly decrease the span of data delivered to the input terminal of the recognition system, since the recognition system is relieved of the task of deciphering these codes and in place of raw or minimally processed data, indices can be used that are received by way of processing input data in accord with, for instance, Livanov-Lebedev’s hypothesis of phase-frequency information coding in the CNS [5], Kaplan-Shishkin’s EEG segmentation [6], an implementation of V.L. Dunin-Barkovsky’s [7] or J. Hoppfield’s [8] hypothesis of functional neural network architecture, etc. We are basically replacing the as-yet-unknown part of the “model of brain function” or “code book” with what results from the work of the recognition system. If we had full knowledge of the coding system, recognition would clearly be unnecessary. Overall, the use of that—the first—method for achieving direct EEG control of a prosthesis involves adjusting the computer system (through the recognition system) to the unalterable limb (or, more widely, body) control system already present in the brain.
The second method of achieving direct electroencephalographic (in a broad sense) control of a prosthesis or computer system is to use arbitrary EEG indices to create a state space for the control system (block 1.2.2, fig. 1). In this case, the person is adapted to the unalterable limb control system. This is typically achieved in the following way: First of all, a system of control inputs is chosen, for instance an increase in the power of the EEG in the alpha range to 02 at 10% represents the signal that triggers the action “raise hand five degrees”, and a decrease triggers the action “lower hand five degrees”. After that, the person is trained to change the chosen parameters at will. There are several different ways of going about doing this:
- Using the technique of biological feedback to train the person to control the dynamics of individual indices or their patterns (block 1.2.2.1, fig. 1). It has long been known that this method can enable a person to exercise control over the functioning of both an individual nerve cell and a complex EEG pattern. It is precisely this method that is typically used for training a person to exercise neural control. Generally EEG spectra that can be tied to various leads (so that the control spaces can be made more complex) are used as control indices. In this method, the signal used for providing feedback is one lacking any vital independent meaning: a picture on a screen, a sound, a light bulb, vibration, etc.
- Using methods based on operant conditioning (block 1.2.2.2, fig. 1). This method is basically very similar to the method of biofeedback, the difference being that the stimulus/feedback possesses its own vital meaning (this is typically the “reward-punishment” system of stimuli). In this method, the linking of a physiological function to a signal—that is, the forming of a new functional system—occurs unconsciously. For obvious reasons, this method is more widely used in work with animals, but this technique can be and is used on people as well—particularly in the near-threshold formation of conditional reflexes for experimental purposes, or for controlling EEG patterns for putting them into a form that matches a positive prognosis when a patient comes out of a coma. In the latter case, the voices of loved ones, for example, are used as a positive stimulus.
In the second method of achieving EEG control of a bionic prosthesis—training an arbitrarily chosen set of EEG parameters connected with a control system—there is a common set of problems, no matter which technique is being used (biofeedback or operant conditioning).
First of all, existing EEG events are used as control stimuli. Their very existence speaks to the fact that they reflect processes that impact the CNS. And when, while they are being trained to control a prosthesis, they are embedded into a new functional system, the system they were in before may suffer. It clearly makes sense to use systems of as low a level as possible—as low a level as individual cells—as control parameters. That will minimize the damage to the system in which the control parameter was originally embedded. However, at present, that method requires either an invasive approach or highly expensive hardware and software. An alternative solution is to use systems that are not rigidly tied to any existing functional systems as control parameters. For example, if you assume that there are neural ensembles that retain the pattern of the result when fulfilling a task and store the alphabet of objects when solving multiple-choice problems, etc., then the use of signals from that kind of ensemble will in no way damage the system as a whole, since it is their very nature to readjust quickly to a given task.
Second of all, those sorts of skills do not automate well—that is, the way any complex skill is learned is that, as the elements of it are practiced and become more predictable, we cease to be aware of them, leaving only the “peak” of the hierarchical functional systems under conscious control. In the case of neurointerfaces, this almost never happens. The reasons for this are not completely clear, though we can surmise that while the control system of a real limb has many levels, in the case of an artificial limb all the internal complexity of the control system is “projected onto the surface”, or reduced to a single level. It would be advisable to test whether it is possible to construct a multi-level artificial-limb control system that uses biofeedback and to automate lower-level skills over the course of the training process. The low informational throughput that is often cited as a complicating factor in using neurointerfaces to solve the practical issues involved in controlling complex artificial systems is, in our view, the result of the same “flattening” of the control system—the notorious ceiling of three bits of information in a signal which can be the address in the state space of the control system, a space that is already linked to a whole range of necessary actions of individual actuators. The information capacity of the control signal in this case clearly depends on the complexity of the control system and the complexity of the corresponding control space.
Thirdly, control of the function is difficult to maintain—more often than not, the system settles at the state of “maximum” or that of “minimum”. In our opinion, at the base of this problem is a lack of feedback from intermediate “control points”. The operator does not have a sense of these points, since he does not receive separate feedback about their transmission. During the training process, he must identify a control strategy capable of isolating the location of the signal on a control point, a strategy that is distinct from the strategy of fitting the signal to the next point. Nonlinear feedback may help in finding the point. Nonlinearity may be achieved in one of two ways. The first way is via a graph that shows the value of the control function in the feedback signal space as, for instance, a parabola, with the control point at the bend in the function. The second way is via a graph of the feedback signal that acts as a function of multiple variables, or is simply a complex signal from the function pattern. It is well known that biofeedback training goes a lot faster when you use function patterns instead of training the operator to control a single specific parameter.
Having thus examined the two main approaches to the task of creating a control system for artificial limbs (or, more broadly, for artificial systems), we would like to point out that the use of pattern recognition systems to identify and predict the positions and locations of control parameters in EEG, etc., are not typically used in conjunction with a biocontrol system that uses biofeedback—though that approach is potentially quite interesting (block 1.3, fig. 1). The reasons are obvious: If both “interlocutors”—the person and the computer—are modulated, then that modulation cannot occur, since it will lack predictability. For example: The recognition system recognizes the pattern of a command, but at the same time the person changes the corresponding pattern, detaching it from the functional system the delivered command is a part of.
Overall, what needs to be done is to build a functional system as quickly as possible that will be responsible for controlling a new limb in a person’s system of mental control. Here’s a simpler way of thinking about the process of constructing this system: the operator, possessing a general outline of the result, begins to act using the new system. The control stimuli in the unfamiliar system lead to haphazard results, chaotic movements. At the next stage, noises are gradually expelled from the system, in a way consistent with Bernstein’s stages of the construction of movement. That is, the first thing that is done is that the system is provided with “muscle” tone and proprioceptive connections are found that indicate the status of the tone. Next come levels of synergies, of ensembles of movements, etc. Essentially, the person usually performs high-level control actions, and a command the limb-control system receives is something like “pick the object up from the table”. At the same time, the person is capable of consciously moving individual fingers of his hand by shifting his attention, directly accessing the same levels of movement control that were once automated, hidden within the structure of the complex movement. The routing system for the various levels of the command hierarchy is hidden within the substrate that supports the arrays of functional systems in the brain responsible for different movements. In order for the skill of controlling an artificial hand with many degrees of freedom to be learned faster, this system needs to be partially transferred to an artificial apparatus. This means that when an artificial hand receives the command “pick the object up from the table”, it must automatically coordinate all the lower levels involved in creating that movement. Moreover, it must do so relying on its own “intelligence” that replaces the natural system’s automated levels of control. At the same time, the human operator must be prepared to send a command directly to, for example, a particular finger of the hand at any given moment. Though a definitive solution to this problem has not yet been found, the groundwork for it has already been laid. The answer may lie in creating a simple control structure that appears to the operator as though it consists of two spaces. The first is the command level—from general actions to control of individual actuators on the micro level, i.e. the choice of a control system (the hand as a whole, the wrist, the shoulder, etc.). The second is a command for a directed action in a space limited by the control system’s degrees of freedom. When a high-level command is sent, the lower levels in the hierarchy are controlled by the computer system (in automated control system mode), which underwent training (in pattern recognition mode) while the operator was mastering these levels of control. As a result, the required overall informational capacity of the control signal can be made incomparably smaller.
We have examined certain key problems connected with the practical task of creating a neuro-controlled whole-body or individual-limb prosthesis and proposed a basic classification of the methods of addressing these issues. These are problems related both to the simulation of living systems when creating an externalized movement-control system and to the required measurement tools in a broad sense—both the actual registration instruments and the basic data-processing tools. The goal of enabling an operator to exercise control of a bionic prosthesis and, more broadly, an artificial body strikes us as an achievable one on the whole.
/ About us
Founded by Russian entrepreneur Dmitry Itskov in February 2011 with the participation of leading Russian specialists in the field of neural interfaces, robotics, artificial organs and systems.
The main goals of the 2045 Initiative: the creation and realization of a new strategy for the development of humanity which meets global civilization challenges; the creation of optimale conditions promoting the spiritual enlightenment of humanity; and the realization of a new futuristic reality based on 5 principles: high spirituality, high culture, high ethics, high science and high technologies.
The main science mega-project of the 2045 Initiative aims to create technologies enabling the transfer of a individual’s personality to a more advanced non-biological carrier, and extending life, including to the point of immortality. We devote particular attention to enabling the fullest possible dialogue between the world’s major spiritual traditions, science and society.
A large-scale transformation of humanity, comparable to some of the major spiritual and sci-tech revolutions in history, will require a new strategy. We believe this to be necessary to overcome existing crises, which threaten our planetary habitat and the continued existence of humanity as a species. With the 2045 Initiative, we hope to realize a new strategy for humanity's development, and in so doing, create a more productive, fulfilling, and satisfying future.
The "2045" team is working towards creating an international research center where leading scientists will be engaged in research and development in the fields of anthropomorphic robotics, living systems modeling and brain and consciousness modeling with the goal of transferring one’s individual consciousness to an artificial carrier and achieving cybernetic immortality.
An annual congress "The Global Future 2045" is organized by the Initiative to give platform for discussing mankind's evolutionary strategy based on technologies of cybernetic immortality as well as the possible impact of such technologies on global society, politics and economies of the future.
Future prospects of "2045" Initiative for society
2015-2020
The emergence and widespread use of affordable android "avatars" controlled by a "brain-computer" interface. Coupled with related technologies “avatars’ will give people a number of new features: ability to work in dangerous environments, perform rescue operations, travel in extreme situations etc.
Avatar components will be used in medicine for the rehabilitation of fully or partially disabled patients giving them prosthetic limbs or recover lost senses.
2020-2025
Creation of an autonomous life-support system for the human brain linked to a robot, ‘avatar’, will save people whose body is completely worn out or irreversibly damaged. Any patient with an intact brain will be able to return to a fully functioning bodily life. Such technologies will greatly enlarge the possibility of hybrid bio-electronic devices, thus creating a new IT revolution and will make all kinds of superimpositions of electronic and biological systems possible.
2030-2035
Creation of a computer model of the brain and human consciousness with the subsequent development of means to transfer individual consciousness onto an artificial carrier. This development will profoundly change the world, it will not only give everyone the possibility of cybernetic immortality but will also create a friendly artificial intelligence, expand human capabilities and provide opportunities for ordinary people to restore or modify their own brain multiple times. The final result at this stage can be a real revolution in the understanding of human nature that will completely change the human and technical prospects for humanity.
2045
This is the time when substance-independent minds will receive new bodies with capacities far exceeding those of ordinary humans. A new era for humanity will arrive! Changes will occur in all spheres of human activity – energy generation, transportation, politics, medicine, psychology, sciences, and so on.
Today it is hard to imagine a future when bodies consisting of nanorobots will become affordable and capable of taking any form. It is also hard to imagine body holograms featuring controlled matter. One thing is clear however: humanity, for the first time in its history, will make a fully managed evolutionary transition and eventually become a new species. Moreover, prerequisites for a large-scale expansion into outer space will be created as well.
Key elements of the project in the future
• International social movement
• social network immortal.me
• charitable foundation "Global Future 2045" (Foundation 2045)
• scientific research centre "Immortality"
• business incubator
• University of "Immortality"
• annual award for contribution to the realization of the project of "Immortality”.